-
chevron_right
Quantum computing progress: Higher temps, better error correction
news.movim.eu / ArsTechnica · Wednesday, 27 March - 22:24 · 1 minute
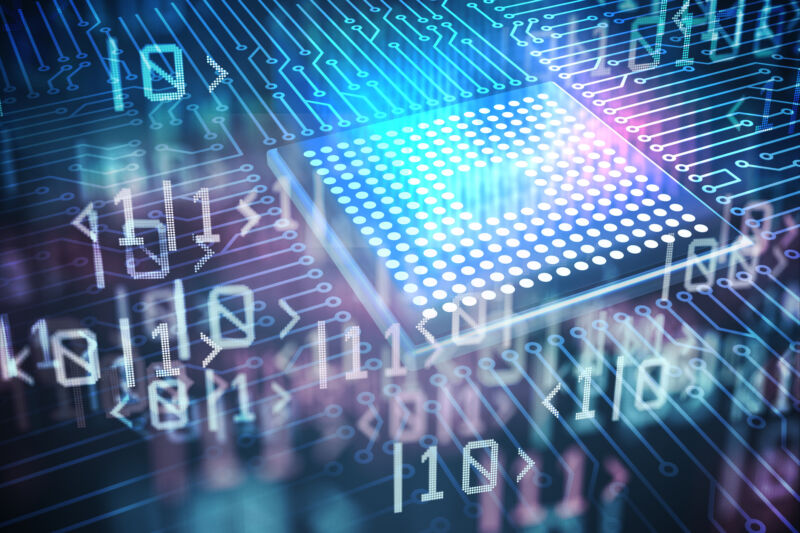
There's a strong consensus that tackling most useful problems with a quantum computer will require that the computer be capable of error correction. There is absolutely no consensus, however, about what technology will allow us to get there. A large number of companies, including major players like Microsoft, Intel, Amazon, and IBM, have all committed to different technologies to get there, while a collection of startups are exploring an even wider range of potential solutions.
We probably won't have a clearer picture of what's likely to work for a few years. But there's going to be lots of interesting research and development work between now and then, some of which may ultimately represent key milestones in the development of quantum computing. To give you a sense of that work, we're going to look at three papers that were published within the last couple of weeks, each of which tackles a different aspect of quantum computing technology.
Hot stuff
Error correction will require connecting multiple hardware qubits to act as a single unit termed a logical qubit. This spreads a single bit of quantum information across multiple hardware qubits, making it more robust. Additional qubits are used to monitor the behavior of the ones holding the data and perform corrections as needed. Some error correction schemes require over a hundred hardware qubits for each logical qubit, meaning we'd need tens of thousands of hardware qubits before we could do anything practical.